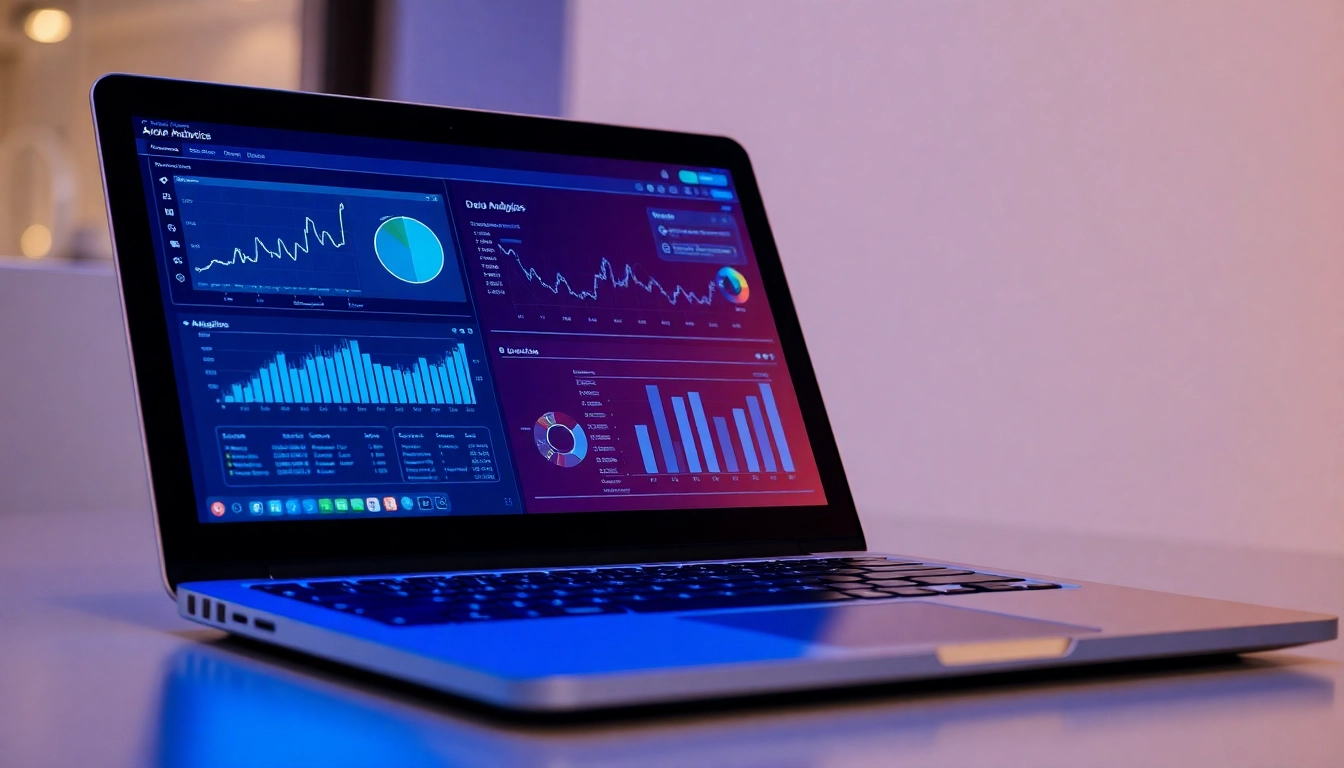
Understanding the Basics of Data Analytics
What is Data Analytics?
Data analytics refers to the systematic computational analysis of data. It involves various processes to inspect, clean, and model data with the aim to discover useful information, inform conclusions, and support decision-making. In a world awash with data, the ability to extract valuable insights is crucial. Businesses, in particular, rely on data analytics to enhance operational efficiency, understand customer behavior, and drive strategic initiatives. Websites like https://www.informaticsview.com offer valuable resources and articles on the subject, helping to expand understanding and application of these concepts.
Key Components of Data Analytics
Understanding data analytics requires insight into its key components, including:
- Data Collection: This involves gathering raw data from various sources, ensuring a comprehensive dataset for analysis. Sources might include transactional data, sensor data, social media, and surveys.
- Data Processing: Collected data needs to be processed and cleaned. This step entails removing duplicates, handling missing values, and transforming data types for analysis.
- Data Analysis: At this stage, various analytical techniques, such as statistical analysis, data mining, and machine learning, are employed to uncover patterns and insights.
- Data Visualization: Visualizing data through charts, graphs, and dashboards makes it easier to interpret results and communicate findings to stakeholders.
- Decision Making: The ultimate goal of data analytics is to inform better decision-making at all levels within an organization.
The Importance of Data in Decision-Making
Data-driven decision-making is paramount in today’s business environment. Organizations using data analytics can achieve:
- Improved Accuracy: With access to data insights, companies can reduce the reliance on intuition alone and make informed decisions based on empirical evidence.
- Enhanced Agility: Real-time data analytics allow businesses to quickly adapt to market changes, customer preferences, and emerging trends.
- Competitive Advantage: Leveraging data can provide a significant edge over competitors by identifying new opportunities for growth and operational efficiencies.
Applying Data Analytics in Your Business
Identifying Your Business Needs
The first step in applying data analytics is to identify the specific needs of your business. This involves understanding key objectives and challenges. Engaging relevant stakeholders is critical to this process. Consider asking questions such as:
- What are the primary goals of the business?
- What challenges are we currently facing?
- What decisions can data analytics help us make?
By identifying these needs, businesses can focus their analytics efforts on relevant areas, such as customer segmentation, marketing campaigns’ effectiveness, or operational efficiencies.
Choosing the Right Analytics Tools
Selecting appropriate analytics tools is vital for effective data analysis. The market offers a range of solutions, each with unique features tailored to different business needs. Key considerations when choosing analytics tools include:
- User-Friendliness: Tools should be intuitive and accessible for users across the organization.
- Integrations: Ensure that the tool can integrate with existing systems and data sources.
- Scalability: Select tools that can grow with your business and handle increased data volumes.
- Analytical Features: Look for features that align with your specific analytical needs, such as predictive analytics, visualization capabilities, and reporting functions.
Popular analytics tools include Google Analytics, Tableau, Microsoft Power BI, and SAS, each offering distinct advantages depending on the use case.
Setting Up Analytics for Success
Establishing a robust analytics framework is essential for success. This involves:
- Data Governance: Implement data governance policies to ensure data integrity, privacy, and compliance with regulations.
- Training and Support: Provide adequate training to staff on analytics tools and encourage a data-driven culture across the organization.
- Defining Metrics: Clearly define KPIs so that everyone understands what success looks like and how it aligns with business objectives.
Common Challenges in Data Analytics
Data Quality Issues
One of the most significant challenges in data analytics is ensuring data quality. Poor quality data can lead to inaccurate insights, resulting in misguided decisions. To address these issues, businesses should:
- Establish robust data collection procedures to minimize errors.
- Regularly audit and clean datasets to remove inaccuracies and duplicates.
- Utilize data profiling techniques to assess data quality and identify areas needing improvement.
Interpreting Data Effectively
Data interpretation can be subjective, especially when faced with complex datasets. To mitigate this, businesses should:
- Encourage diverse viewpoints in data analysis to avoid bias and enrich understanding.
- Utilize data visualization tools to represent insights clearly, making analysis easier for non-analytical stakeholders.
- Regularly communicate and discuss insights within teams to ensure alignment.
Integrating Analytics into Business Processes
Successfully integrating analytics into existing business processes can be challenging. Companies should consider the following strategies:
- Embed analytics into daily operations by creating dashboards that provide real-time insights.
- Foster collaboration between data teams and business units to ensure analytics initiatives align with business goals.
- Solicit feedback from users on analytics processes to refine and improve them over time.
Advanced Data Analytics Techniques
Predictive Analytics Explained
Predictive analytics involves using statistical algorithms and machine learning techniques to identify the likelihood of future outcomes based on historical data. This powerful technique is applicable across various industries, including finance for fraud detection, healthcare for patient outcomes, and retail for inventory management. Key steps in implementing predictive analytics include:
- Identifying relevant historical data that indicates potential future outcomes.
- Choosing appropriate predictive modeling techniques, such as regression analysis, decision trees, or neural networks.
- Validating and refining models based on performance metrics.
- Continuously updating models with new data to improve predictive power.
Leveraging Machine Learning for Insights
Machine learning, a subset of artificial intelligence, has transformed data analytics by providing algorithms that enable systems to learn from data and improve over time. Businesses can leverage machine learning for tasks such as:
- Automating data processing and analysis.
- Enhancing customer segmentation by identifying more nuanced patterns in consumer behavior.
- Developing recommendation engines that personalize user experiences.
To get started with machine learning, businesses should ensure they have access to high-quality data, the necessary computational resources, and expertise in data science.
Visualizing Data for Better Communication
Effective data visualization is crucial for communicating insights derived from data analytics. Good visualization helps in:
- Simplifying complex data to highlight important points.
- Engaging stakeholders and facilitating discussions around data findings.
- Improving retention of information by presenting it in a visually appealing format.
Common data visualization tools include Tableau, Power BI, and Google Data Studio, which offer functionalities to create interactive dashboards and visuals tailored to diverse audiences.
Measuring the Success of Data Analytics Initiatives
Key Performance Indicators to Track
To assess the effectiveness of data analytics initiatives, businesses should define and measure relevant KPIs, such as:
- Customer Acquisition Cost (CAC): Understanding the cost associated with acquiring new customers helps evaluate marketing effectiveness.
- Return on Investment (ROI): Calculating the ROI of analytics projects can determine their financial impact and justify continued investment.
- Data Accuracy Metrics: Regularly measuring the accuracy of data can help improve data quality processes and reduce errors.
Analyzing ROI of Analytics Investments
Calculating ROI for analytics investments involves assessing both the financial and operational impact of the initiatives. This can be executed by:
- Calculating the direct cost savings or revenue increases attributed to analytics projects.
- Evaluating time savings to quantify increased operational efficiencies.
- Using case studies and past project outcomes to make projections for future analytics investments.
Continuous Improvement through Feedback
Continuous improvement is fundamental to ensuring analytics initiatives remain effective and relevant. Businesses should implement feedback loops where:
- Data users provide insights on the usability and relevance of analytics tools and reports.
- A/B testing is employed to assess the effectiveness of different data strategies.
- Analytics teams continuously adapt their methodologies in response to shifting business needs.